News
How Adversarial ML Can Turn An ML Model Against Itself
Discover the main types of adversarial machine learning attacks and what you can do to protect yourself.
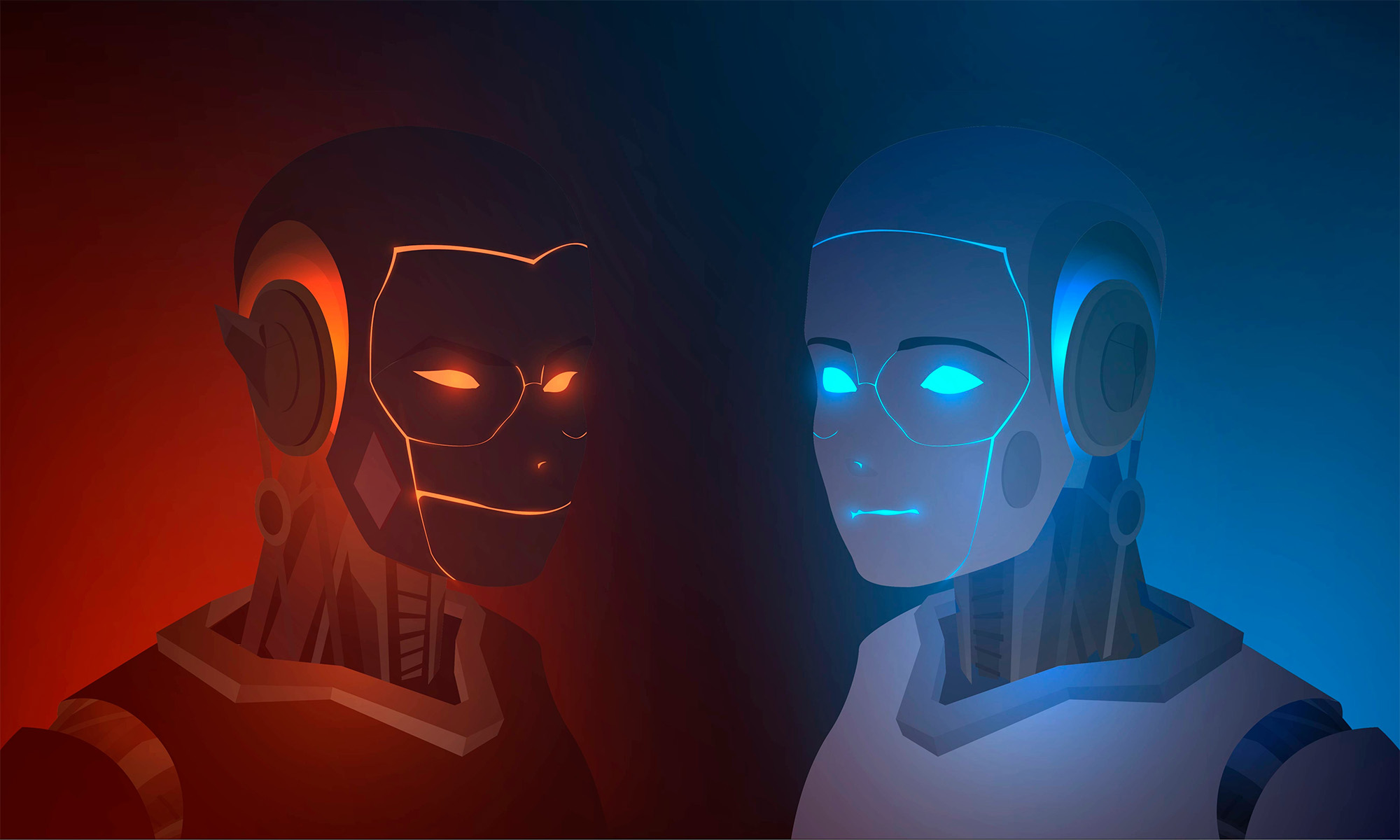
Machine learning (ML) is at the very center of the rapidly evolving artificial intelligence (AI) landscape, with applications ranging from cybersecurity to generative AI and marketing. The data interpretation and decision-making capabilities of ML models offer unparalleled efficiency when you’re dealing with large datasets. As more and more organizations implement ML into their processes, ML models have emerged as a prime target for malicious actors. These malicious actors typically attack ML algorithms to extract sensitive data or disrupt operations.
What Is Adversarial ML?
Adversarial ML refers to an attack where an ML model’s prediction capabilities are compromised. Malicious actors carry out these attacks by either manipulating the training data that is fed into the model or by making unauthorized alterations to the inner workings of the model itself.
How Is An Adversarial ML Attack Carried Out?
There are three main types of adversarial ML attacks:
Data Poisoning
Data poisoning attacks are carried out during the training phase. These attacks involve infecting the training datasets with inaccurate or misleading data with the purpose of adversely affecting the model’s outputs. Training is the most important phase in the development of an ML model, and poisoning the data used in this step can completely derail the development process, rendering the model unfit for its intended purpose and forcing you to start from scratch.
Evasion
Evasion attacks are carried out on already-trained and deployed ML models during the inference phase, where the model is put to work on real-world data to produce actionable outputs. These are the most common form of adversarial ML attacks. In an evasion attack, the attacker adds noise or disturbances to the input data to cause the model to misclassify it, leading it to make an incorrect prediction or provide a faulty output. These disturbances are subtle alterations to the input data that are imperceptible to humans but can be picked up by the model. For example, a car’s self-driving model might have been trained to recognize and classify images of stop signs. In the case of an evasion attack, a malicious actor may feed an image of a stop sign with just enough noise to cause the ML to misclassify it as, say, a speed limit sign.
Model Inversion
A model inversion attack involves exploiting the outputs of a target model to infer the data that was used in its training. Typically, when carrying out an inversion attack, an attacker sets up their own ML model. This is then fed with the outputs produced by the target model so it can predict the data that was used to train it. This is especially concerning when you consider the fact that certain organizations may train their models on highly sensitive data.
How Can You Protect Your ML Algorithm From Adversarial ML?
While not 100% foolproof, there are several ways to protect your ML model from an adversarial attack:
Validate The Integrity Of Your Datasets
Since the training phase is the most important phase in the development of an ML model, it goes without saying you need to have a very strict qualifying process for your training data. Make sure you’re fully aware of the data you’re collecting and always make sure to verify it’s from a reliable source. By strictly monitoring the data that is being used in training, you can ensure that you aren’t unknowingly feeding your model poisoned data. You could also consider using anomaly detection techniques to make sure the training datasets do not contain any suspicious samples.
Secure Your Datasets
Make sure to store your training data in a highly secure location with strict access controls. Using cryptography also adds another layer of security, making it that much harder to tamper with this data.
Train Your Model To Detect Manipulated Data
Feed the model examples of adversarial inputs that have been flagged as such so it will learn to recognize and ignore them.
Perform Rigorous Testing
Keep testing the outputs of your model regularly. If you notice a decline in quality, it might be indicative of an issue with the input data. You could also intentionally feed malicious inputs to detect any previously unknown vulnerabilities that might be exploited.
Adversarial ML Will Only Continue To Develop
Adversarial ML is still in its early stages, and experts say current attack techniques aren’t highly sophisticated. However, as with all forms of tech, these attacks will only continue to develop, growing more complex and effective. As more and more organizations begin to adopt ML into their operations, now’s the right time to invest in hardening your ML models to defend against these threats. The last thing you want right now is to lag behind in terms of security in an era when threats continue to evolve rapidly.
News
Samsung Smart Glasses Teased For January, Software Reveal Imminent
According to Korean sources, the new wearable will launch alongside the Galaxy S25, with the accompanying software platform unveiled this December.
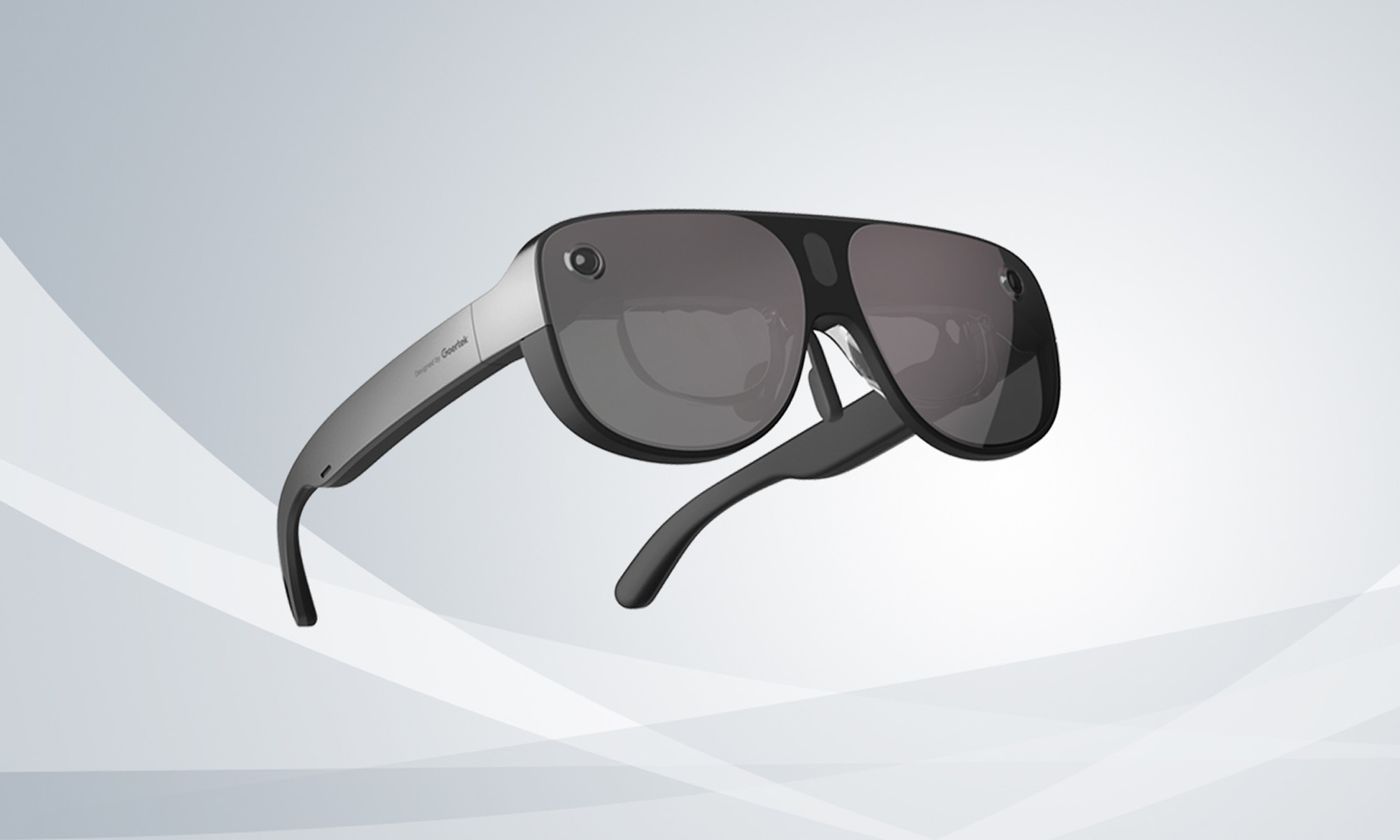
Samsung appears poised to introduce its highly anticipated smart glasses in January 2025, alongside the launch of the Galaxy S25. According to sources in Korea, the company will first reveal the accompanying software platform later this month.
As per a report from Yonhap News, Samsung’s unveiling strategy for the smart glasses echoes its approach with the Galaxy Ring earlier this year. The January showcase won’t constitute a full product launch but will likely feature teaser visuals at the Galaxy S25 event. A more detailed rollout could follow in subsequent months.
Just in: Samsung is set to unveil a prototype of its augmented reality (AR) glasses, currently in development, during the Galaxy S25 Unpacked event early next year, likely in the form of videos or images.
Additionally, prior to revealing the prototype, Samsung plans to introduce…
— Jukanlosreve (@Jukanlosreve) December 3, 2024
The Galaxy Ring, for example, debuted in January via a short presentation during Samsung’s Unpacked event. The full product unveiling came later at MWC in February, and the final release followed in July. Samsung seems to be adopting a similar phased approach with its smart glasses, which are expected to hit the market in the third quarter of 2025.
A Collaborative Software Effort
Samsung’s partnership with Google has played a key role in developing the smart glasses’ software. This collaboration was first announced in February 2023, with the device set to run on an Android-based platform. In July, the companies reiterated their plans to deliver an extended reality (XR) platform by the end of the year. The software specifics for the XR device are expected to be unveiled before the end of December.
Reports suggest that the smart glasses will resemble Ray-Ban Meta smart glasses in functionality. They won’t include a display but will weigh approximately 50 grams, emphasizing a lightweight, user-friendly design.
Feature Set And Compatibility
The glasses are rumored to integrate Google’s Gemini technology, alongside features like gesture recognition and potential payment capabilities. Samsung aims to create a seamless user experience by integrating the glasses with its broader Galaxy ecosystem, starting with the Galaxy S25, slated for release on January 22.